Idun cluster upgrading
Upgrading infrastucture is critical to meet AI demands
The powerful Idun cluster is upgrading to new AI workloads
The Idun compute cluster at NTNU is the most powerful GPU-system in academia in Norway, making it well suited for advanced AI-workloads. Idun is now preparing to deal with the challenges forwarded by the rapid research developments in artificial intelligence.
-The ambitious plans for a Norwegian generative language model among them, calls for even larger investments. I am happy to announce that a joint effort between the Department of Computer Science, NorwAI, The Faculty of Information Technology and Electrical Engineering and the IT Division at NTNU have joined hands in an effort to further increase the capacity and capabilities of IDUN, making it better suited for developing large models, says Arne Dag Fidjestøl, Head of IT Operations Section at NTNU IT Division.
-Without this effort, we would be unable to meet the ambitious plans for NorwAI, he adds.
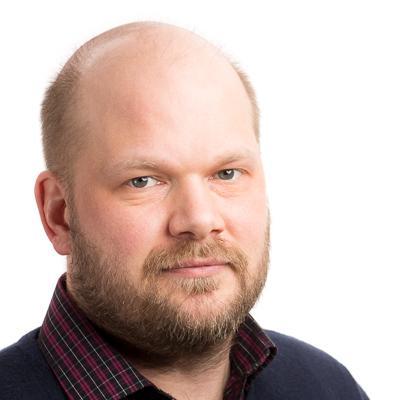
The funding will be spent on upgrading the IDUN cluster, which is the name of the hybrid GPU/CPU-cluster already in place at NTNU.
The first NorwAI NorGPT-models have been running on Idun for four months to complete the initial build-up of the current NorGPT-23 (23 billion parameters). Parameters are the model’s ability to express how fine nuances are captured in the language’s orthography, syntax, semantics, and pragmatics. The number of words from different sources is more than 18 billion.
NorGPT-40
The next step in the NorGPT-series is the 40 billion editions to be set up this coming winter. If successful, the NorGPT-40 will be operational in 2024. NorwAI is right now driving a national effort to build an alternative to the Californian language models that will easily dominate these new domains if nobody takes on the challenge. The consequences for Norwegian language, Norwegian digital independency and innovation possibilities for industry could be fatal.
Upgrading the national infrastructures are critical steppingstones to be prepared for the quantum leap technology now are facing.

Norwegian language models NorGPT.
Photo: Kai T. Dragland
A 4-step process
Building language models is a four-step process. First, you need to collect the textual data. The texts come from different sources and need to be processed to ensure high quality.
Second, the model is trained on a large computer cluster, such as NTNU’s Idun. The training runs through all texts and adjusts the model to predict masked parts correctly.
Third, the model is fine-tuned. Fine-tuning enables the model to execute a set of desired functions. For instance, the model can be fine-tuned to generate formal language, write poems, or summarize longer texts.
Fourth, the model produces output which is presented to humans. The humans’ feedback is used to align the model. Alignment ensures that the model responds to requests in a desired way.
The IDUN cluster
IDUN is the formal name of the GPU/HPC cluster already in place at NTNU. It plays a key role in fields such as artificial intelligence, unconventional computing, nano systems as well as more traditional HPC workloads like computational fluid dynamics (CFD).
Idun is a system provided by the IT Division that aims at providing a high-availability and professionally administrated computer platform for NTNU. All researchers at NTNU can get a base access to the system in order to do smaller computations or test the system for suitability, but in order to make use of substantial resources the user need to be associated by a shareholder in the system which give them access to a certain capacity. It is an effort to combine the computer resources of individual shareholders to create a cluster for rapid testing and prototyping of HPC software.
While the IT division provides the backbone of the cluster, such as switches for high-speed interconnection, storage, and provisioning servers, the individual faculties/departments finance the computer resources. Any faculty or department can become a shareholder in the cluster by financing computer capacity, leveraging their share of computer time as well as the computer time of other idling resources. Accounting guarantees each partner's share of computer time and ensures fairness between the users on the system.

Increased demand
The AI and machine learning research community at NTNU is growing at a fast rate and the number of research projects and collaboration agreements with external partners are increasing rapidly. This calls for more powerful computing resources, with secure and efficient handling of data shared between NTNU researchers and external partners as a key element. NTNU has invested heavily in running upgrades of the system, resulting in substantial yearly investments since 2017.
Parts of the funding for greater capacity will be spent on new software and hardware that will improve the functionality for secure storage of large quantities of data. This will improve the ability of the infrastructure to store and process data from external partners, with a particular focus on sensitive data.
Sensitive data
There are several risks related to handling sensitive data, such as unauthorized disclosure and data breaches. Secure storage and processing of data is indeed essential in several ongoing projects and is a necessity for collaborations with a variety of partners. Streamlining data storage and processing (e.g., for the purpose of building machine learning models) will make sharing of business sensitive data easier between external partners and NTNU researchers and students.
A further goal
A final goal behind the investment is to enable quality visualization of data and results. Visualization plays an important role when developing several machine learning pipelines, especially when dealing with sensitive data, and we would like to see more of this at NTNU.
By: Rolf D. Svendsen, NorwAI
PUBLISHED 2023-08-11